Machine Learning Seminar: Likelihood-based Inference for Stochastic Epidemic Models with application to High-resolution Contact Tracking Data
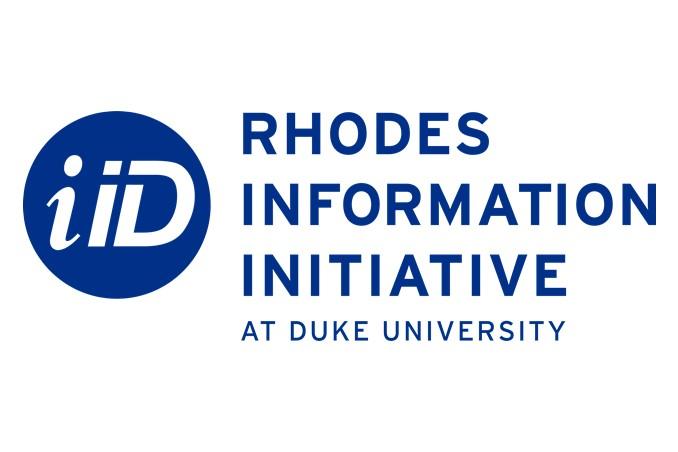
Stochastic epidemic models such as the Susceptible-Infectious-Removed (SIR) model are widely used to model the spread of disease at the population level, but fitting these models poses significant challenges when missing data or latent variables are present. In particular, the likelihood function has long been considered intractable in such settings. We will discuss our recent work using generating function techniques that newly enable likelihood computations without model simplifications in the presence of missing infection and recovery times. Motivated by a study of influenza transmission with social contact tracking data, we then present a data-augmented MCMC algorithm for fitting parameters of the SIR model when the underlying contact network evolves continuously. This co-evolution entails interdependence between individuals' disease statuses and social contacts through time. We demonstrate that accounting for the contact network and epidemic dynamics jointly is crucial for valid inference, and apply the method to analyze data from the eX-FLU study of influenza on a college campus.