Hierarchical Structures in Bayesian Statistics
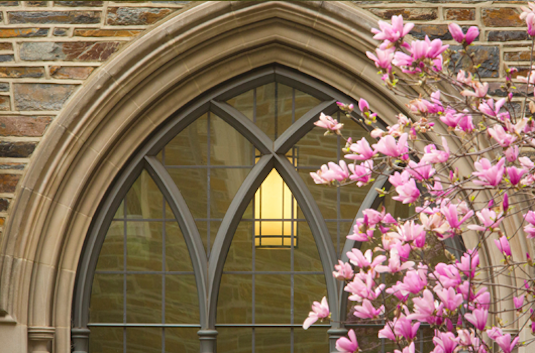
In this talk I will give an overview of my two main lines of research, Bayesian nonparametric modelling and theory of Bayesian computation, by discussing hierarchical models, widely applied probabilistic structures that allow to borrow information among distinct groups.
Particular emphasis will be given on the inferential and computational implications of this specification, starting from applied examples.
In the second part of the talk I will focus on the study of Gibbs samplers, which are popular algorithms to approximate posterior distributions arising from Bayesian models. Despite their popularity and good empirical performances, however, there are still relatively few quantitative theoretical results on their scalability or lack thereof, e.g. much less than for gradient-based sampling methods. In a work with Giacomo Zanella (Bocconi University), we introduce a novel technique to analyse the asymptotic behaviour of mixing times of Gibbs Samplers, based on tools of Bayesian asymptotics. Our methodology applies to high-dimensional regimes where both number of datapoints and parameters increase, under random data-generating assumptions. The framework is applied to two-level hierarchical models with generic likelihoods and exponential family priors. In this context we are able to provide dimension-free convergence results for Gibbs Samplers under mild conditions.