Learning differential equation models from noisy biological data
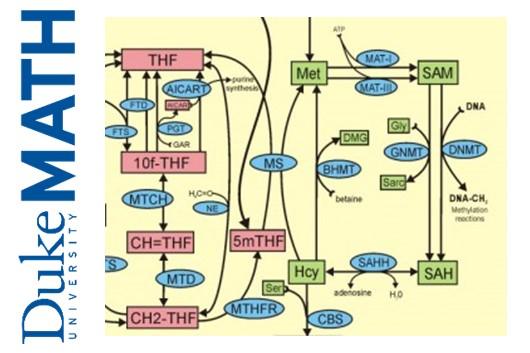
Equation learning aims to discover differential equations that describe the underlying dynamics of time series data. Biological systems have unique challenges in the application of equation learning, namely, the observed data are sparsely measured and have high levels of proportional error noise. Moreover, equation learning methods can suffer from the model specification problem in which the governing differential equation model must be specified a priori either explicitly or as a library of candidate terms. This poses a challenge for biological systems, which are often described by nonlinear terms with unknown exponents. These issues help explain why equation learning has seen very few applications to real-world biological systems. In this talk, I will discuss methods that were developed to overcome these challenges and present, to the best of my knowledge, the first successful application of equation learning to real biological data. The methodology is demonstrated on simulated data of biological transport from the Fisher-KPP equation and experimental data of collective cell migration in scratch assays.