Sampling Multi-modal Distributions using Simulated Tempering Langevin Monte Carlo
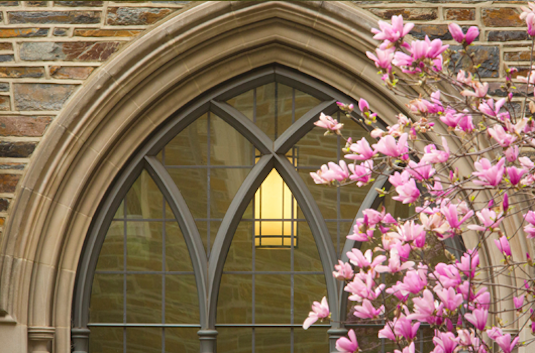
A fundamental problem in Bayesian statistics is sampling from distributions that are only specified up to a partition function (constant of proportionality). In particular, we consider the problem of sampling from a distribution given access to the gradient of the log-pdf. For log-concave distributions, classical results due to Bakry and Emery show that natural continuous-time Markov chains called Langevin diffusions mix in polynomial time. But in practice, distributions are often multi-modal and hence non-log-concave, and can take exponential time to mix.
We address this problem by combining Langevin diffusion with simulated tempering. The result is a Markov chain that mixes in polynomial rather than exponential time by transitioning between different temperatures of the distribution. We prove fast mixing for any distribution that is close to a mixture of gaussians of equal variance.
For the analysis, we bound the spectral gap using a novel Markov chain decomposition theorem. Previous approaches rely on decomposing the state space as a partition of sets, while our approach can be thought of as decomposing the stationary measure as a mixture of distributions (a "soft partition").
Based on the paper https://arxiv.org/abs/1812.00793
Joint work with Rong Ge (Duke) and Andrej Risteski (MIT).